AI CDSS
Ex-Vivo 조건에서 공초점 형광현미경으로
획득한 영상 데이터로 세포를 암/염증/정상
상태로 분류할 수 있는 딥러닝 모델 개발을
진행하고 있습니다.
제한된 데이터를 극복하기 위하여 전이학습(Transfer learning)
및 데이터 확장(Data augmentation)기술을 적용하고 있습니다.
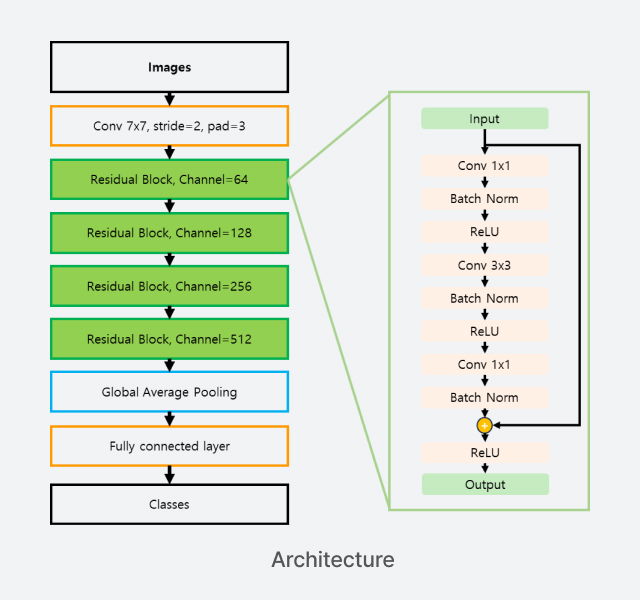
3종의 Machine learning-based method과 비교하여 개발된 딥러닝 모델의 우수성을 확인하였고, 계속하여 알고리즘을 개선 중에 있습니다.
Machine Learning and deep learning performance
Methods | Accuracy | FPR | FNR | Precision | Recall | F1-score |
---|---|---|---|---|---|---|
XGB | 0.7495 | 0.1401 | 0.2578 | 0.7591 | 0.7423 | 0.7488 |
SVM | 0.6981 | 0.1673 | 0.3195 | 0.7191 | 0.6806 | 0.6786 |
RF | 0.7058 | 0.1656 | 0.3285 | 0.7196 | 0.6717 | 0.6886 |
Proposed | 0.8173 | 0.0964 | 0.2408 | 0.8186 | 0.7588 | 0.7784 |
Qualitative Results
Test data samples (a) and the results of class activation map (b).
Neoplasm examples are shown in the top row, inflammation in the middle row,
and normal in the bottom row, respectively.
Red arrows on neoplasm images indicate the area where the
glands are hard to see, and yellow arrows on normal images indicate
where the glands are uniformly represented.
The arrangement of gland is an important feature on neoplasm
classification task, and it can be seen that CAM focuses well on this area.
The white bars on the lower right of the image indicates the scale 50 μm.
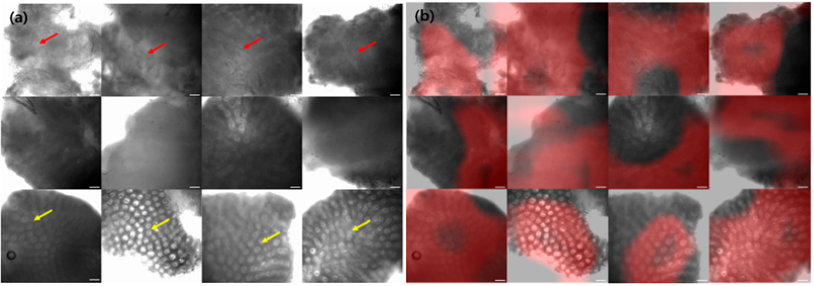